The Importance of Data Quality
- Audiit
- Apr 9
- 4 min read
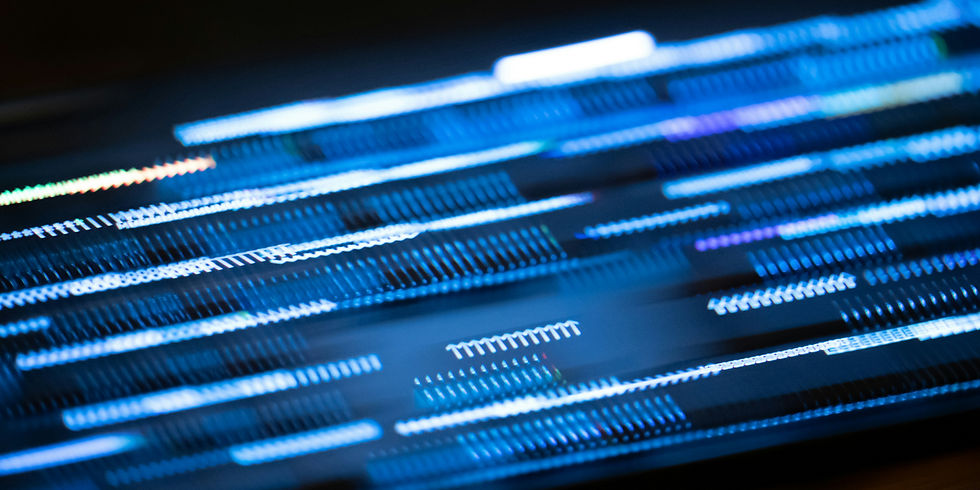
In the previous blogs, we explored the power of project data and the transition from regular reporting to advanced analytics. Now, let’s delve into a crucial aspect that underpins all data-driven initiatives: data quality. For stakeholders in the infrastructure industry, such as planners, schedulers, PMO leaders, and senior corporate managers, understanding and ensuring high-quality data is paramount to maximizing the value of Enterprise Project Portfolio Management (EPPM) data.
Data Quality Fundamentals
What Constitutes High-Quality Data?
High-quality data is accurate, complete, consistent, and timely. These attributes ensure that the data can be reliably used for analysis and decision-making. Here’s a closer look at each attribute:
Accuracy: Data must correctly represent the real-world entities it describes. Inaccurate data can lead to flawed analyses and poor decision-making.
Completeness: All necessary data should be present. Missing data can create gaps in analysis, leading to incomplete insights.
Consistency: Data should be uniform across different datasets and systems. Inconsistencies can cause confusion and errors in data interpretation.
Timeliness: Data should be up-to-date and available when needed. Outdated data can result in decisions based on obsolete information.
Examples of data quality impact on analytics
Aspect of Data Quality | Impact on Analytics | Example |
Accuracy | Incorrect insights and decisions | Misreported project costs leading to budget overruns |
Completeness | Incomplete analysis and missed opportunities | Missing project timelines causing delays in project delivery |
Consistency | Conflicting data leading to confusion | Different versions of project status reports causing misalignment |
Timeliness | Outdated insights and delayed decisions | Using old project data resulting in irrelevant forecasts |
Relevance | Irrelevant data leading to wasted resources | Analyzing data that does not impact project outcomes |
Reliability | Untrustworthy insights and lack of confidence | Inconsistent project performance metrics causing mistrust |
Impact on Analytics
How Poor Data Quality Can Derail Analytics Efforts
Poor data quality can significantly hinder the effectiveness of analytics. Here are some ways it can impact your projects:
Misleading Insights: Inaccurate or incomplete data can lead to incorrect conclusions, affecting project outcomes and strategic decisions.
Increased Costs: Cleaning and correcting poor-quality data requires additional resources, increasing project costs.
Reduced Efficiency: Inconsistent data can slow down analysis processes, reducing overall efficiency and productivity.
Eroded Trust: Stakeholders may lose confidence in the data and the decisions based on it, leading to resistance to data-driven initiatives.
Best Practices for Ensuring Data Quality
Ensuring Data Accuracy, Completeness, and Consistency
To maintain high data quality, infrastructure organizations should adopt the following best practices:
Data Governance Framework: Establish a robust data governance framework that defines data quality standards, roles, and responsibilities. This framework should include policies for data entry, validation, and maintenance.
Regular Data Audits: Conduct regular audits to identify and rectify data quality issues. Deploy data monitoring solutions that provide access to transactional history. Audits and real-time monitoring help ensure that data remains accurate, complete, and consistent over time.
Data Quality Tools: Utilize data quality tools that automate the process of detecting and correcting errors. These tools can help maintain data integrity and reduce manual efforts.
Training and Awareness: Educate employees about the importance of data quality and provide training on best practices for data entry and management. Awareness programs can help foster a culture of data quality within the organization.
Data Integration: Ensure seamless integration of data from various sources. Consistent data formats and standards across systems can prevent discrepancies and improve data quality.
Real-Time Monitoring: Implement real-time monitoring systems to detect and address data quality issues as they arise. This proactive approach can prevent minor issues from escalating into major problems.
Case Study: Improving Data Quality in a Large Infrastructure Project
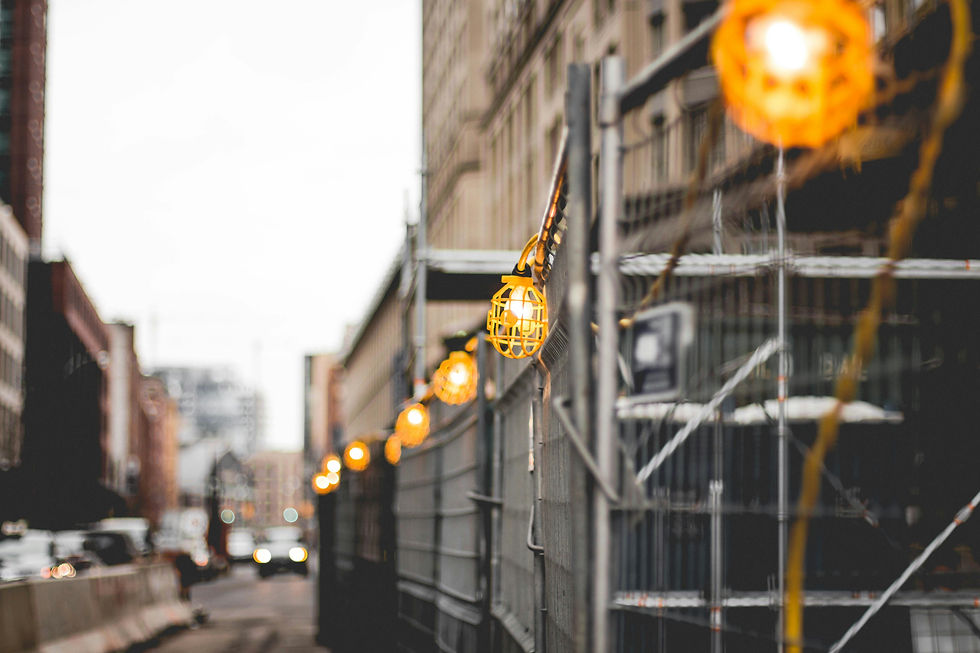
Background
A large engineering and construction firm faced challenges with data quality in its EPPM system. Inaccurate and inconsistent data led to project delays and cost overruns.
Solution
The firm implemented a comprehensive data quality management program, including:
Establishing a data governance framework.
Deploying real-time monitoring tools, that provide transactional history of all changes.
Conducting regular data audits.
Using data quality tools for automated error detection.
Training employees on data quality best practices.
Results
The firm saw significant improvements in project performance, including:
Reduced Project Delays: Accurate and consistent data enabled better project planning and execution, reducing delays by 10% to 15%.
Cost Savings: Improved data quality reduced the need for rework and corrections, resulting in 10% in cost savings.
Enhanced Decision-Making: Reliable data provided better insights, leading to faster and more informed decisions and improved project outcomes.
Encouraging Stakeholders to Prioritize Data Quality
Why It Matters
For planners, schedulers, PMO leaders, and senior corporate managers, prioritizing data quality is essential for maximizing the value of EPPM data. High-quality data enables better project performance, improved returns, and a competitive edge in the infrastructure industry.
Action Steps
Assess Current Data Quality: Evaluate the current state of your data quality and identify areas for improvement.
Implement Best Practices: Adopt the best practices outlined above to ensure data accuracy, completeness, and consistency.
Foster a Data-Driven Culture: Encourage a culture that values data quality and continuous improvement. Engage stakeholders at all levels to support data quality initiatives.
By focusing on data quality, infrastructure organizations can unlock the full potential of their EPPM data, leading to better project performance and improved returns. In the next blog, we will explore the importance of data availability and accessibility, and how to overcome common challenges in this area. Stay tuned!
Comments